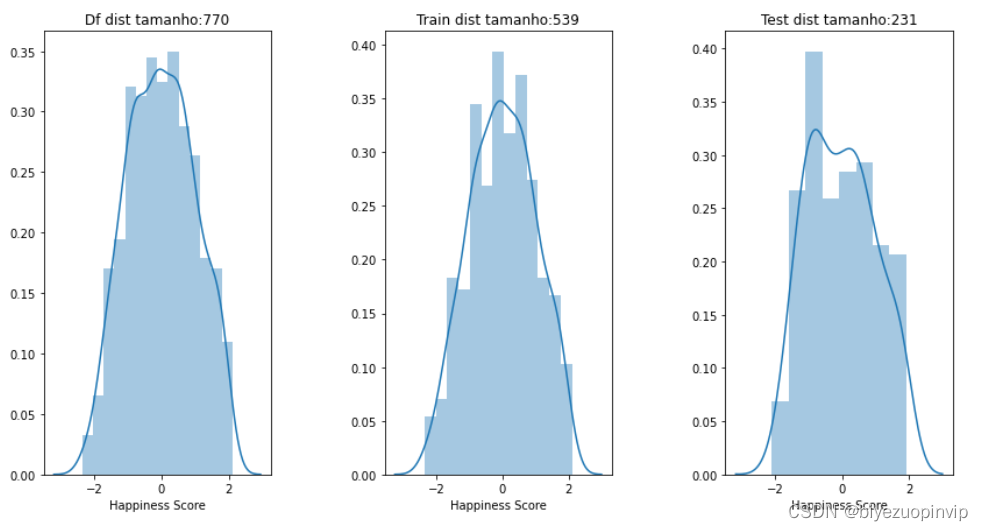
基于Python的世界各个国家的幸福度的公开数据集的数据挖掘
目录一. 问题背景 1二. 准备工作 2三. 具体实施 21.数据存储及基本加载 2。
目录
一. 问题背景 1
二. 准备工作 2
三. 具体实施 2
1.数据存储及基本加载 2
数据整理到数组之中 2
2.数据清洗 2
① 多余列清除 2
② 列属性归一 3
③ 空值处理 4
3.数据挖掘算法 6
① 基本特征 6
② 总体热力图分析 7
③ 对指定数据的热力图分析 8
④ 离散图分析 9
⑤ 点线图分析经济与健康横向对比 10
⑥ 地区划分得到幸福度指数 12
⑦ 深究每个地区的最大因素: 15
四. 总结汇总 16
一.问题背景
《世界幸福报告》是对全球幸福状况的一次里程碑式的调查。第一份报告于2012年发布,第二份于2013年发布,第三份于2015年发布,第四份报告于2016年更新。3月20日,在联合国举行的庆祝国际幸福日的活动上,根据155个国家的幸福水平,发布了《2017年世界幸福》。随着各国政府、组织和民间社会越来越多地使用幸福指数来指导其决策,该报告继续得到全球的认可。经济学、心理学、调查分析、国家统计、卫生、公共政策等领域的权威专家描述了如何有效地利用幸福感来评估国家的进步。这些报告回顾了当今世界的幸福状况,并展示了新的幸福科学如何解释个人和国家在幸福方面的差异。
二.准备工作
小组选择的公开数据集为kaggle上的公开数据集Factors affecting happiness,即一个关于各个国家的幸福度的公开数据集,从2015-2019每一年都有一个数据集。列出了各个国家的幸福度指数,经济情况,健康,宗教信仰,家庭等。
三.具体实施
1.数据存储及基本加载
1)原理分析
通过os库提供的getcwd方法返回当前工作目录,后面跟上每个数据集的相对路径,得到每个数据集的路径。而为open方法指定路径以及编码方式(此处为utf-8)即可获取到数据集文件。同样地,pandas库也为我们提供了专为csv文件提供的读取方法read_csv。为了后续的方便整理和调用,这里将每一年的数据全部放到一个数组之中,通过对下标的引用即可获取到数据集。
本文转载自:http://www.biyezuopin.vip/onews.asp?id=16524
import matplotlib.pyplot as plt
# 定义matplotlib的字体
# plt.rcParams['font.sans-serif'] = ['Droid Sans Fallback']
# boxstyle为文本框的类型,sawtooth是锯齿形,fc是边框线粗细,也可写作 decisionNode={boxstyle:'sawtooth',fc:'0.8'}
decisionNode = dict(boxstyle="round", fc="0.8")
# 定义决策树的叶子结点的描述属性
leafNode = dict(boxstyle="circle", fc="0.8")
# 定义决策树的箭头属性
arrow_args = dict(arrowstyle="<-")
plt.rcParams['font.sans-serif'] = ['KaiTi']
# nodeTxt为要显示的文本,centerPt为文本的中心点,箭头所在的点,parentPt为指向文本的点
def plotNode(nodeTxt, centerPt, parentPt, nodeType):
createPlot.ax1.annotate(nodeTxt, xy=parentPt, xycoords='axes fraction',
xytext=centerPt, textcoords='axes fraction',
va="bottom", ha="center",
bbox=nodeType, arrowprops=arrow_args)
# 获取叶节点的数目
def getNumLeafs(myTree):
# 定义叶子结点数目
numLeaf = 0
# 得到根据第一个特征分类的结果
nodes = myTree.child_nodes
# 遍历得到的子节点
for node in nodes:
# 如果node为一个决策树结点,非子节点
if node.child_nodes:
# 则递归的计算nodes中的叶子结点数,并加到numLeafs上
numLeaf += getNumLeafs(node)
else:
numLeaf += 1
# 返回求的叶子结点数目
return numLeaf
# 获取树的层数
def getTreeDepth(myTree):
# 定义树的深度
maxDepth = 0
# 得到第一个特征分类的结果
nodes = myTree.child_nodes
for node in nodes:
# 如果node为一个决策树结点
if node.child_nodes:
thisDepth = 1 + getTreeDepth(node)
# 如果node为一个决策树结点,非子节点
else:
# 则将当前树的深度设为1
thisDepth = 1
# 比较当前树的深度与最大数的深度
if thisDepth > maxDepth:
maxDepth = thisDepth
# 返回树的深度
return maxDepth
# 绘制中间文本
def plotMidText(cntrPt, parentPt, txtString):
# 求中间点的横坐标
xMid = (parentPt[0] - cntrPt[0]) / 2.5 + cntrPt[0]
# 求中间点的纵坐标
yMid = (parentPt[1] - cntrPt[1]) / 2.5 + cntrPt[1]
# 绘制树结点
createPlot.ax1.text(xMid, yMid, txtString)
# 绘制决策树
def plotTree(myTree, parentPt, nodeTxt):
# 定义并获得决策树的叶子结点数
numLeafs = getNumLeafs(myTree)
# 得到第一个特征
firstStr = myTree.keyword
# 计算坐标,x坐标为当前树的叶子结点数目除以整个树的叶子结点数再除以3,y为起点
cntrPt = (plotTree.xOff + (1.0 + numLeafs) /
len(myTree.child_nodes) / plotTree.totalW, plotTree.yOff)
# 绘制决策树结点,也是当前树的根结点
if parentPt == (0, 0):
parentPt = cntrPt
plotMidText(cntrPt, parentPt, nodeTxt)
plotNode(firstStr, cntrPt, parentPt, decisionNode)
# 根据第一个特征找到子节点
nodes = myTree.child_nodes
# 因为进入了下一层,所以y的坐标要变 ,图像坐标是从左上角为原点
plotTree.yOff = plotTree.yOff - 1.0 / plotTree.totalD
# 遍历字节带你
for node in nodes:
# 如果node为一棵子决策树,非叶子节点
if node.child_nodes:
# 递归的绘制决策树
plotTree(node, cntrPt, node.parent)
# node为叶子结点
else:
# 计算叶子结点的横坐标
plotTree.xOff = plotTree.xOff + 1.0 / plotTree.totalW
# 绘制叶子结点
plotNode(node.keyword, (plotTree.xOff,
plotTree.yOff), cntrPt, leafNode)
# 特征值
plotMidText((plotTree.xOff, plotTree.yOff), cntrPt, node.parent)
# 计算纵坐标
plotTree.yOff = plotTree.yOff + 1.0 / plotTree.totalD
# 主函数 绘图
def createPlot(inTree):
# 定义一块画布
fig = plt.figure(1, facecolor='white')
# 清空画布
fig.clf()
# 定义横纵坐标轴,无内容
axprops = dict(xticks=[], yticks=[])
# 绘制图像,无边框,无坐标轴
createPlot.ax1 = plt.subplot(111, frameon=False, **axprops)
# plotTree.totalW保存的是树的宽
plotTree.totalW = float(getNumLeafs(inTree))
# plotTree.totalD保存的是树的高
plotTree.totalD = float(getTreeDepth(inTree))
# 决策树起始横坐标
plotTree.xOff = -0.5 / plotTree.totalW
# 决策树的起始纵坐标
plotTree.yOff = 1.0
# 绘制决策树
plotTree(inTree, (0, 0), '')
# 显示图像
plt.savefig('tree.jpg')
更多推荐
所有评论(0)